Rebellion Research, a New York-based hedge fund, applies proprietary machine-learning based artificial intelligence technology to rate equities, fixed income, currencies and commodities in 44 countries. Rebellion Research does not engage in high-frequency trading.
“The number one value of machine learning as applied to algorithmic trading is the ability to consider multiple factors and to change weightings on those factors,” said Alexander Fleiss, chief investment officer at Rebellion Research. “Our machine-learning algorithms incorporate both fundamental and economic data. We monitor the global economy and predict outperformance due to mispricings.”
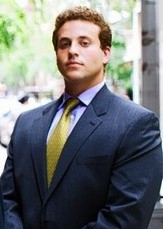
Alexander Fleiss, Rebellion Research
Rebellion Research’s machine learning-based technology estimates the 1-month return for thousands of assets, as well as a confidence level regarding whether each asset will outperform the Fed Fund rate for that month. The assets are then automatically ranked based on these predictions, and a letter grade is assigned to each asset.
“A” ratings are reserved for assets that exhibit both a high-expected return along with a high probability of outperformance, while “F” ratings are given to those assets that have low expected return and a low probability of outperformance. Approximately 10% of assets are assigned an “A” rating, and 10% an “F” rating, but with considerable variation from month to month.
In a historical simulation looking at ratings assigned from 2007 – 2012, a portfolio purchasing all “A” rated assets each month and short selling all “F” rated assets returned 8% annualized. What’s more, the baskets of “A” assets had lower volatility than the baskets of “F” assets over this period. On average, these “A” rated assets were found to have both superior performance and preferable risk characteristics.
Fleiss and Spencer Greenberg, Rebellion Research’s CEO, collaborated on the original machine-based learning concept beginning in 2004 and had a finished product by 2007. Greenberg is currently pursuing a doctorate at The Courant Institute of Mathematical Sciences.
Separately, new research shows that machine-based algorithms may be responsible for “ultra-fast extreme events,” or UEEs, in financial market equity prices.
The study, which was co-authored by Eric Hunsader, CEO of Nanex, provider of a high-performance ticker plant that delivers streaming market data.
Analyzing millisecond-scale data for the world’s largest and most powerful techno-social system, the global financial market, the study reveals an abrupt transition to a new all-machine phase characterized by large numbers of sub second extreme events.
“The proliferation of these sub-second events shows an intriguing correlation with the onset of the system-wide financial collapse in 2008,” according to the study. “Our findings are consistent with an emerging ecology of competitive machines featuring ‘crowds’ of predatory algorithms, and highlight the need for a new scientific theory of sub-second financial phenomena.”
To carry out their research, the investigators assembled a high-throughput millisecond-resolution price stream across multiple stocks and exchanges using the Nanex NxCore software package. They uncovered an explosion of UEEs starting in 2006, just after new legislation came into force that made high frequency trading more attractive.
The resulting data comprises 18,520 UEEs between 2006 and 2011, which are shown visually on the Nanex website at www.nanex.net. These UEEs are of interest from the basic research perspective of understanding instabilities in complex systems, as well as from the practical perspective of monitoring and regulating global markets populated by high frequency trading algorithms, the study said.