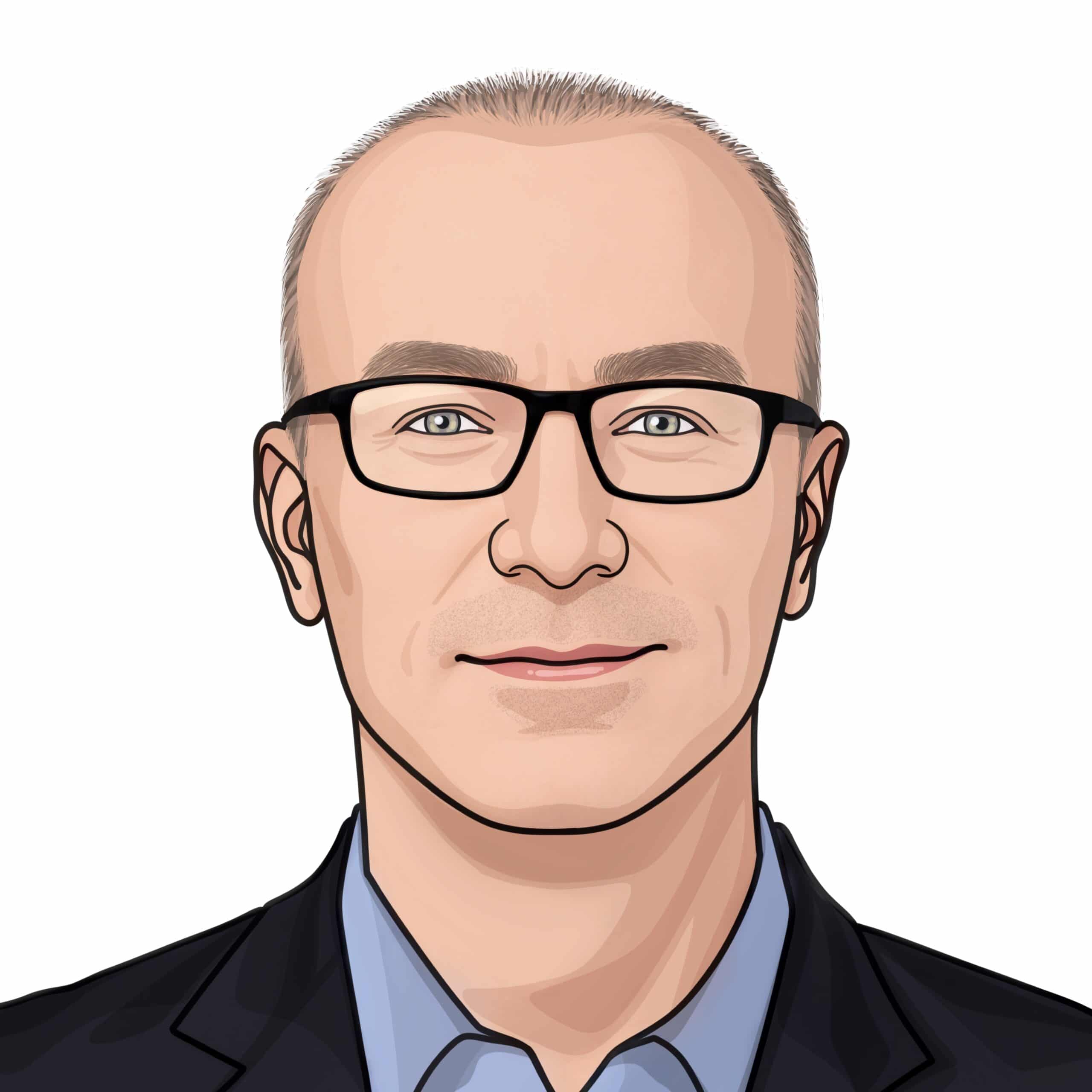
Complex event processing is rapidly being integrated into capital markets applications such as trading and market surveillance, providing an ability to sift through data to uncover patterns.
“Real-time technologies, like CEP, have proven themselves in trading, risk and compliance,” said Dr. John Bates, chief technology officer of Software AG. “Now, they are starting to evolve into complete in-memory analytic compute platforms that offer a combination of analytics for past, present and future scenarios — and that can proactively respond as well as recommend.”
CEP is very different from “business intelligence,” says Bates. “It’s not really BI that people are looking for — that’s very old style ‘operating in the rear view mirror.’ It’s more about what we call “Intelligent Business Operations” — which is about real-time continuous intelligence — and thus you have the ability to respond when you detect the wrong thing is about to happen or there is an opportunity to take advantage of an opportunity.”
The advent of high-frequency trading has given an undeniable push to CEP middleware software systems, according to research firm Research and Markets.
“The traditional Business Intelligence (BI) tools require structured data and relational databases to process and provide strategic outputs,” Research and Markets said in a report. “As well, the realization of opportunity is delayed as the process of analyzing databases takes a huge amount of time. Therefore, there was a need of high velocity detection and responsive technology. This led to the creation of better value proposition for organizations to innovate and improvise event based technologies.”
CEP is not just about decision support — which implies a human is in the loop,” Bates said. “Sometimes it is also about “sense and respond” – i.e., taking an automated intelligent action.”
One example is self-learning trading algorithms. Some firms are using self-learning real-time techniques to tune in to new evolving pattern, such as correlating thousands of different market movements against other market movements to look for new correlations and trends. When they are found, the system can self-generate algorithms to mine them and then self-tuning the parameters to fit the most profitable combination.
“If you know what to trade then you can build an algorithm to look for the pattern and then place orders,” Bates said. “However, finding the golden patterns as they flash into and out of existence over days, weeks, months or years is complex. Some innovative firms are using these real-time techniques. It’s more than traditional predictive analytics because instead of being based on past data and being batch-oriented in nature, it is real-time and continuous – so recommendations and actions can be taken immediately – yielding first-mover advantage.”
Another example is moving trading surveillance and risk to real-time — spotting an emerging threat – such as a rogue trader – by baselining over time what is “normal trading behavior” and then spotting a trader who is behaving unusually.
“An example could be a trader that has placed an unusual, large trade, which is then followed in moments by a new article that moves the market by 25% in that instrument,” Bates said. “Was this an insider trade or a lucky trade? Was it correlated with messaging network traffic or phone activity? Has the trader been working unusual hours? Is it part of a pattern? Analyzing this in real-time means that we can act before it is too late.”