As with any cutting-edge technology, artificial intelligence-based trading systems—incorporating machine learning, neural networks and other AI stratagems—offer limitless potential but are not without risk.
The business case for applying machine-based learning to trading algorithms is intuitive, compelling and measurable.
“Today, connections between institutions, brokers and trading venues are electronic, so you have all the moving components in electronic format,” said Alfred Eskandar, chief executive of trading system provider Portware. “All you need to analyze that is computing power, which is now in ample supply.”
Portware’s Alpha Vision is an “algorithmic optimization” system that uses predictive analytics to automatically select and implement the optimal execution strategy.
“Alpha Vision’s predictive analytics and algorithmic optimization technology acts as a dynamic feedback look for traders,” said Eskandar.
The system automates the process of analyzing orders, monitoring market conditions, selecting the appropriate algorithmic strategy and implementing the optimal execution schedule.
By dynamically switching between algorithms, Alpha Vision eliminates information leakage about large orders, protecting firms from the impact of high-frequency trading.
Neural Networks
A branch of artificial intelligence called neural networks is being used to replicate the intelligence of a human trader.
Neural networks are trainable algorithms that emulate the work of the human brain. This gives them self-training ability to formalize unclassified information and provide analysis of historical data.
“We have a great opportunity to use neural networks in trading,” said Alex Phelps, vice-president of business development at software vendor ETNA Software, in a blog posting. “The neural network receives the data provided by you or some market data feed and analyzes it. After the analysis is over, you receive the output data with a forecast of the possible performance of the asset in the future.”
ETNA Software provides ETNA Robot, an algorithmic trading platform which allows users to trade securities of any class.
ETNA Robot supports HFT with up to 5,000 orders per second. It has a distributed architecture, which means each server runs its own strategy, and is a broker-neutral solution and can be integrated with any broker or market data feed, the company says.
The greatest advantage of neural networks, Phelps said, “is that you can perform analysis of the forecast after some time passes and you receive historical data of its performance as well. Then you can start all over again.”
There’s a downside, however. The application of neural networks to trading is relatively new. Because of that, it’s not proven that neural networks can analyze historical data perfectly with the expected outcome.
“The main disadvantage of neural nets is that they rely solely on the historical performance of the asset, and it is entirely impossible to foresee some events which can greatly affect the market,” said Phelps.
Also, regardless of graphical user interfaces, some neural networks use complex mathematical formulas and this can reduce the functionality neural networking.
“According to the opinion of some financial professionals, the market is a complex structure and it is impossible to learn all its trends,” said Phelps. “Provided that it is impossible for a human being to make accurate predictions, artificial intelligence which imitates a human brain cannot do it, either.”
Human Intuition
While machines can be programmed to think like humans, they lack human intuition.
“Algorithms are still not as smart as experienced humans,” said John Bates, chief technology officer at software firm Progress Software. “I’ve seen hedge funds that are set up to trade with AI algorithms that claim to guarantee they know the market will go up or down tomorrow. These funds have often closed down because they didn’t get it quite right and things changed quickly.”
Spencer Greenberg, whose Rebellion Research hedge fund employs machine learning strategies, says that while there’s much hype surrounding AI, true practitioners are few and far between, and that products that are marketed as AI-based may only succeed at obtaining the wrong answer faster.
“There are a lot of ways to do machine learning wrong,” said Greenberg, who is pursuing a doctorate at New York University’s Courant Institute of Mathematical Sciences. “You can download off-the-shelf machine learning algorithms and come to false conclusions as a result of overfitting the data.”
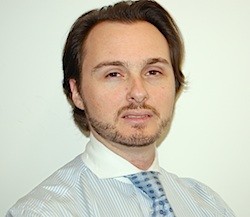
Tim Grant, Benchmark Solutions
The ability to draw generalizations from data may in fact be the gating factor of AI-based trading strategies.
“The obvious pitfall is undemanding what the machine’s telling you,” said Bruce Bland, head of algorithmic research at trading technology firm Fidessa. “If you’re crunching 20-dimensional vectors, you might not be able to discern a pattern. Once you’ve found a particular pattern, you can ask the machine to look for similar ones, but the challenge lies in understanding the data that you’re analyzing.”
Noted Tim Grant, head of marketing and sales at Benchmark Solutions, a provider of streaming market data for fixed income: “These can be very hard problems to solve and in many cases there’s a great deal of research and development that needs to go into finding commercially viable solutions. Also, having the right data feeds is crucial to a successful outcome, and they can be very expensive.”