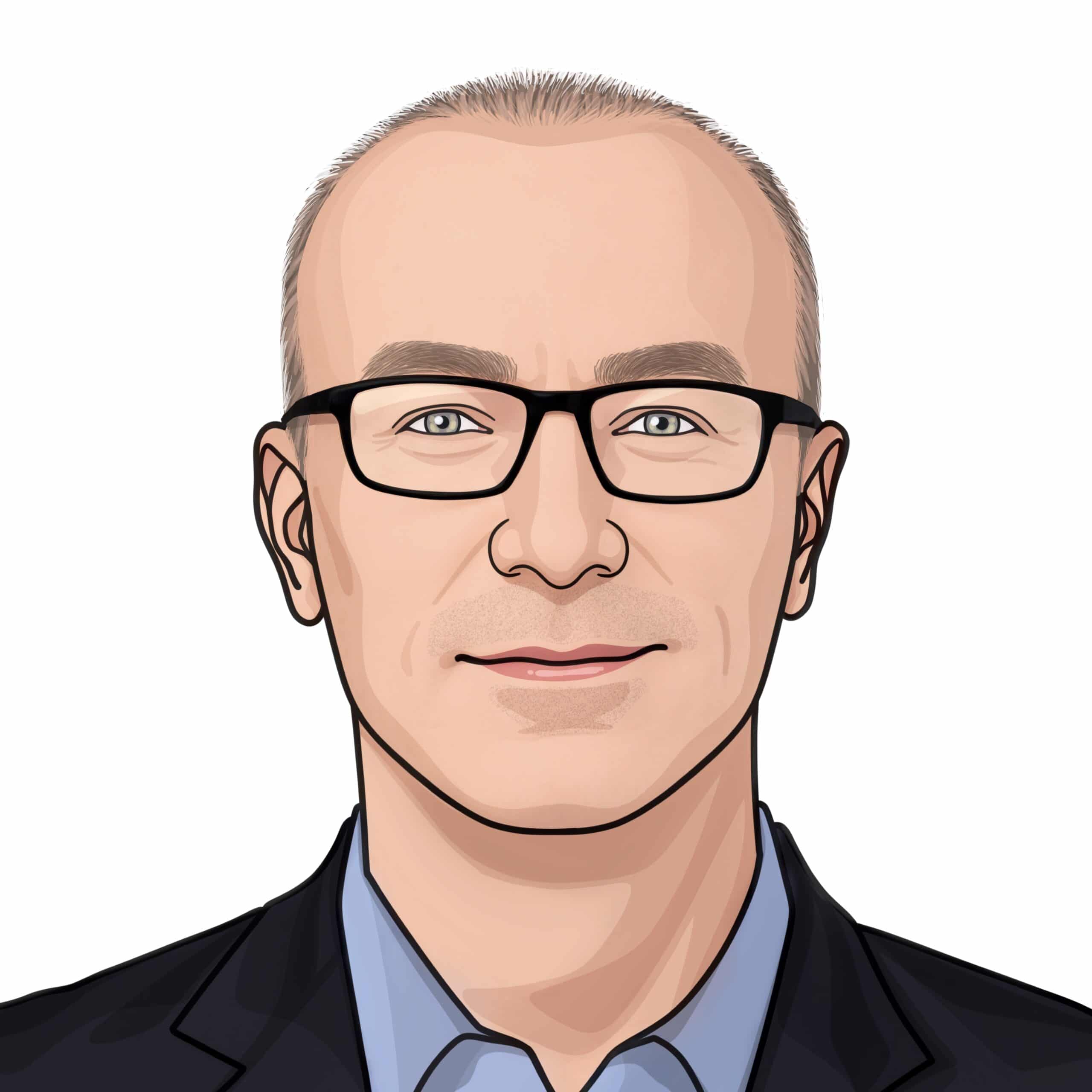
 
‘Big data’ is defined as extremely large data sets that can be analyzed computationally to reveal patterns, trends, and associations.
Data doesn’t get any bigger than on today’s institutional trading desks, where scores of buy and sell orders are transmitted, cancelled and executed every second, across dozens of trading venues, in rapid-fire electronic markets.
A trading and investment firms doesn’t stand a chance of harnessing these torrents of data with Excel spreadsheets and keystrokes.
“You can’t do all this in real-time manually, so it’s about automating the analytics around the decision-making process the traders do with every order,” said Curt Engler, head of U.S. equities trading at J.P. Morgan Asset Management. “Taking data and distilling it down to lead to actions.”
Automation itself is old hat — today’s cutting edge is about evolving the automation by baking intelligence, or machine learning capabilities, into the process.
Larger investment managers have more at stake when it comes to optimizing automation on the trading desk, simply because they have more transactions to monitor — and glean insights from. With $1.75 trillion in total assets, J.P. Morgan was the world’s sixth-largest asset manager as of year-end 2014, according to P&I/Towers Watson.
As Engler explained to Markets Media, automating the analytics on his desk — which trades $230 billion in U.S. stocks across all capitalizations — is an ongoing effort.

Curt Engler, J.P. Morgan
Phase one was automating smaller, retail-sized orders. Phase two was systematizing the trading process, i.e. trying to manage the distribution of outcomes by developing a schedule for the algorithm to automatically place the child orders — marketable and non-marketable, lit or dark — out in the market.
Phase three is adding more electronic block trading capabilities to our systematic processes. Phase four — which is in its early stages — is systematizing strategy selection and broker selection, based on historical and real-time data.
“Now that we have much more name-specific data on market share, whether it’s ATS (Alternative Trading System) or TRF (Trading Reporting Facility) non-ATS data, we can start seeing market share both in the market data that we collect and on our own orders,” Engler said. “This historical data can start guiding us to where the liquidity was. We’re also collecting FIX (Financial Information eXchange) tags real-time, to try to ascertain where the liquidity is.”
Using inputs such as order size, market conditions, and the style of the portfolio manager, the machine advises on how to proceed.
“That’s phase four – arming the trader with as much data as possible to help with the decision-making process by giving a recommendation that’s distilled automatically,” Engler said. “We have a lot of work to do on this, but we have collected an enormous amount of data.”
“This is our next frontier as far as automating the data collection on a macro level, and then reconnaissance — trying to find out what strategy to use and where to go,” he continued. “It’s a multi-year effort, and then to think even bigger-picture, you’ll be able to automate that process even more.”
As data can be dense, one way to better understand its significance — and maximize the benefit of next-generation analytics — is to present it visually.
“We can capture the entire blotter and analyze every single order, giving back to the trader a recommended execution horizon, an execution strategy, and a rating for every single order before they do anything,” said Alfred Eskandar, chief executive officer of trading-technology provider Portware, a FactSet company. “Couple that with our visualization module, which enables the trader to see this analysis in interactive and dynamic charts rather than a wall-of-numbers blotter, and you have a compounded benefit of machine learning analysis coupled with visualization.”
The objective is to enable a trader to draw on empirical support to make critical decisions in the shortest time possible. “A trader should never have to go out on the ledge and ‘just trust the software’,” Eskandar said. “We feel that he or she needs to have concrete evidence and proof behind every decision, because we live in a world where everything is scrutinized.”
Previously in this series:
Featured image by sakkmesterke/Adobe Stock