With algorithmic trading firmly entrenched in the electronic equity landscape, buy-side traders on an eternal quest for alpha preservation are moving beyond algo selection to algo optimization, which entails monitoring and calibrating as the trade is going down.
“The real objective is to get best execution, which often requires not only picking an algorithm but managing the parameters of that algorithm subject to market conditions,” said Michael Earlywine, head trader, North America at $1.2 billion asset manager Ecofin.
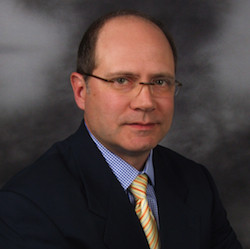
Michael Earlywine, Ecofin
Earlywine cites a golf analogy: selecting the right club for a given shot is necessary, but it’s just one step toward hitting the best shot. A player also needs to use the right blend of strength and finesse, as well as drive it straight or to the left or right as the situation warrants. “Algorithms are like the clubs in your bag,” he said. “Once you pick a club, you still have to swing it correctly. If the green slopes left to right, you need to make sure you hit it so the ball doesn’t bounce off. That’s changing the parameters.”
Added Earlywine, “it’s choosing an algo and using it like the tool that it is, as opposed to saying ‘I picked VWAP (Volume-Weighted Average Price) and it didn’t work.’”
With many investment firms deploying algorithms provided by sell-side brokers, any edge in trading using algorithms necessitates that traders manage them, either directly or in collaboration with their broker.
“One does have to intelligently manage algos in the buy-side trading context,” said Milind Sharma, CEO at QuantZ Capital Management. “Clearly, at this point the basic offerings are fairly commoditized and mainstream and there’s de minimis differentiation between the major algo providers given that even the newer generation algo offerings have converged for the most part.”
“The buy-side trader can add value in terms of execution alpha through the judicious choice of algo parameters,” Sharma said.
While algos fall under the category of machine, it’s evident that man is still needed to optimize the machines, increasingly so in some instances. “It’s the trader’s responsibility to use those tools in a responsible, proactive manner,” Earlywine said. “Today, in many cases, the algos are on their desk and they are actually going in and manipulating the parameters…Having a tool like a VWAP algo and changing the parameters based on market conditions is the only responsible way to use that algo.”
Earlywine works with sell-side providers to optimize algorithms. “My job is to coerce and enforce the brokers into using their own algorithms properly by making them change parameters, by questioning their choice of algos and how they are performing.”
Algorithms can be tweaked by using the data they throw off in the course of generating orders, according to developers and practitioners.
“In the last year or so we’ve seen quite a bit of interest from firms which would like to use metrics from monitoring systems,” said Kevin Covington, CEO of ITRS Group. “For example, metrics show how the technology stack is performing, venue availability and which venue provides the lowest latency. An institution might choose where to direct its trade flow based on that information.”
By creating a feedback loop mechanism between algorithms and order flow, institutions can make algorithm performance more predictable, and therefore more efficient.
“Algorithm performance is predictable as a function of order flow metrics, which means there’s an opportunity to improve performance by leveraging that predictability, by using algorithms that are expected to perform well given the observed order flow,” said Henri Waelbroeck, global head of research at trading systems provider Portware.
For a buy-side trader deploying algos, the objective is to manage the process “by setting control parameters and adjusting them as needed,” Waelbroeck said. “Predictive control is a method for turning data into an optimal algorithm management policy. Algorithm management involves choosing a style (such as dark, participation or stealth) and setting several other control parameters within the same style, such as the participation rate or aggression.”
Keith Saxton, head of financial services at IBM Research, notes the applicability of cognitive computing to the world of algorithmic trading. Cognitive computing refers to the development of computer systems modeled after the human brain.
“There are ways of using predictive technology that enable you to pre-program and have algorithms to learn to be more efficient,” Saxton said. “If you think of how cognitive technology and predictive analytics and machine learning can be applied to algorithms, you’re into a new game.”